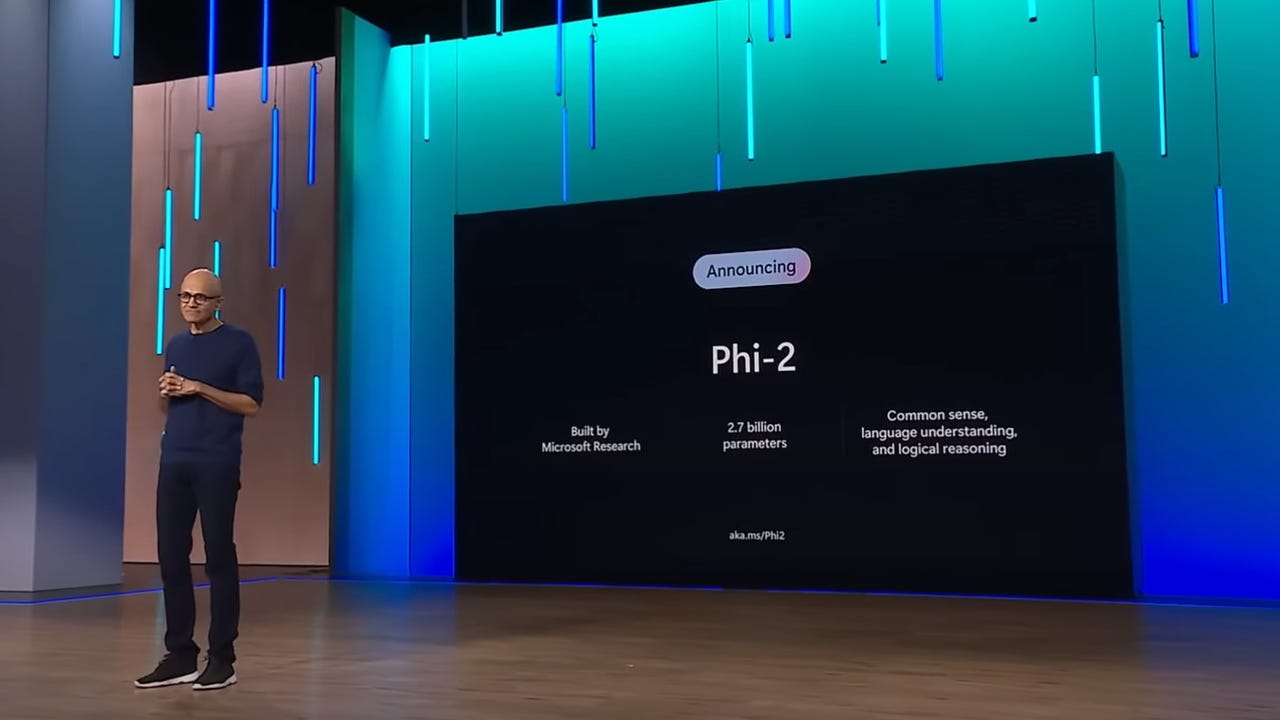
When you think of language models in relation to generative artificial intelligence (AI), the first term that probably comes to mind is large language model (LLM). These LLMs power most popular chatbots, such as ChatGPT, Bard, and Copilot. However, Microsoft’s new language model is here to show that small language models (SLMs) have great promise in the generative AI space, too.
On Wednesday, Microsoft released Phi-2, a small language model capable of common-sense reasoning and language understanding, and it’s now available in the Azure AI Studio model catalog.
Also: AI in 2023: A year of breakthroughs that left no human thing unchanged
Don’t let the word small fool you, though. Phi-2 packs 2.7 billion parameters in its model, which is a big jump from Phi-1.5, which had 1.3 billion parameters.
Despite its compactness, Phi-2 showcased “state-of-the-art performance” among language models with less than 13 billion parameters, and it even outperformed models up to 25 times larger on complex benchmarks, according to Microsoft.
Also: Two breakthroughs made 2023 tech’s most innovative year in over a decade
Phi-2 outperformed models — including Meta’s Llama-2, Mistral, and even Google’s Gemini Nano 2, which is the smallest version of Google’s most capable LLM, Gemini — on several different benchmarks, as seen below.
Phi-2’s performance results are congruent with Microsoft’s goal with Phi of developing an SLM with emergent capabilities and performance comparable to models on a much larger scale.
Also: ChatGPT vs. Bing Chat vs. Google Bard: Which is the best AI chatbot?
“A question remains whether such emergent abilities can be achieved at a smaller scale using strategic choices for training, e.g., data selection,” said Microsoft.
“Our line of work with the Phi models aims to answer this question by training SLMs that accomplish performance on par with models of much larger scale (yet still far from the frontier models).”
When training Phi-2, Microsoft was very selective about the data used. The company first used what it calls “text-book quality” data. Microsoft then augmented the language model database by adding carefully selected web data, which was filtered on educational value and content quality.
So, why is Microsoft focused on SLMs?
Also: These 5 major tech advances of 2023 were the biggest game-changers
SLMs are a cost-effective alternative to LLMs. Smaller models are also useful when they are being used for for a task that isn’t demanding enough to necessitate the power of an LLM.
Furthermore, the computational power required to run SLMs is much less than LLMs. This reduced requirement means users don’t necessarily have to invest in expensive GPUs to power their data-processing requirements.