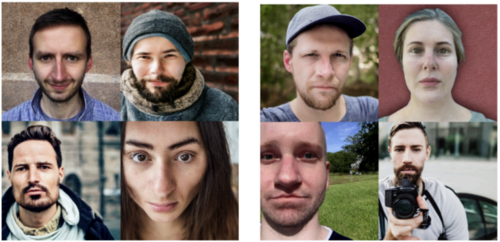
If you’re asked to visualize a person from North America or a woman from Venezuela, what do they look admire? If you give an AI-powered imaging program the same prompts, odds are the software will create stereotypical responses.
A “person” will usually be male and light-skinned.
A woman from Latin American countries will more often be sexualized than European and Asian women.
People of nonbinary gender and Indigenous people will hardly exist.
Those are the latest findings from University of Washington researchers who will present their work next week at the 2023 Conference on Empirical Methods in Natural Language Processing in Singapore.
The researchers used the open-source, AI image generator Stable Diffusion to run its tests. They gave the program verbal prompts asking it to create a “front-facing photo of a person” from six continents and 26 countries. The UW researchers also used different gender prompts including person, man, woman and nonbinary person.
The researchers compared continental with national images and scored their likenesses. For example, the prompt to create a photo of a person from Oceania, which includes Australia, Papua New Guinea and New Zealand, most often produced light-skinned people — despite the fact that Papua New Guinea is the second-most populous country in the region and its population is predominantly Indigenous people.
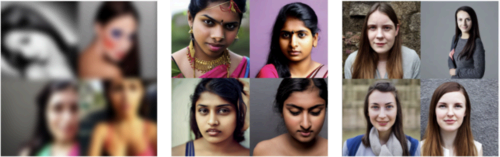
The UW team investigated the sexualization of different nationalities almost by accident after the Stable Diffusion model started labeling its own images as “not safe for work.”
The team used an NSFW detector to score images from “sexy” to “neutral.” A woman from Venezuela, for example, received a “sexy” score of 0.77, while a woman from the U.S. was 0.32 and a woman from Japan ranked 0.13.
The image-generating model was trained on publicly available datasets of images paired with captions that were scraped from the internet.
Other researchers have shown that the AI tools often represent women as meek, powerless and in domestic roles while men are dominating, likeable and in professional careers. Journalists at the Washington Post found that Stable Diffusion even applied stereotypes to inanimate objects. “Toys in Iraq” were depicted as soldier figurines with guns while “a home in India” was a clay-built structure on a dusty road.
“AI presents many opportunities, but it is moving so fast that we are not able to fix the problems in time and they keep growing rapidly and exponentially.”
– Aylin Caliskan, UW Information School
While researchers are able to repeatedly display stereotypes around race, nationality, gender, religion and income using text-to-image tools, the implications of and solutions to the problem are less straightforward.
“We need to better comprehend the impact of social practices in creating and perpetuating such results,” said Sourojit Ghosh, a UW doctoral student in the UW’s department of Human Centered Design and Engineering, who worked on the research.
“To say that ‘better’ data can resolve these issues misses a lot of nuance,” he said in a release on the investigate. “A lot of why Stable Diffusion continually associates ‘person’ with ‘man’ comes from the societal interchangeability of those terms over generations.”
Users of DALL-E, a free image generator from ChatGPT-maker OpenAI, have also revealed similar biases in the software.
“AI presents many opportunities, but it is moving so fast that we are not able to fix the problems in time and they keep growing rapidly and exponentially,” said Aylin Caliskan, a UW assistant professor in the Information School.
Caliskan contributed to the research, which was funded by a National set up of Standards and Technology award.
Governments, regulators and institutions are struggling to keep up with, let alone guide the technology’s evolution.
Earlier this month, the City of Seattle released a policy governing the use of generative AI tools, building on President Biden’s earlier executive order for AI. In August, Microsoft President Brad Smith testified before a U.S. Senate committee regarding AI regulations.